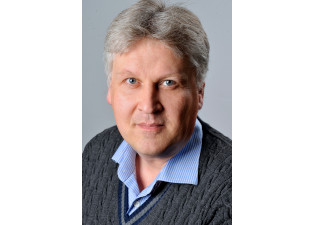
07.02.2024 - Distinguished Lecture Series: Sepp Hochreiter (Johannes Kepler Universität Linz)
We are pleased to announce our upcoming Distinguished Lecture Series talk by Sepp Hochreiter (Johannes Kepler Universität Linz)! The talk will take place in person on February 7th, in room UN32.101. Professor Hochreiter will also be available for meetings on February 6th and on February 7th. If you are interested in scheduling a meeting, please email .
Sepp Hochreiter is heading the Institute for Machine Learning, the LIT AI Lab and the AUDI.JKU deep learning center at the Johannes Kepler University of Linz. Sepp Hochreiter is a pioneer of Deep Learning. His contributions the Long Short-Term Memory (LSTM) and the analysis of the vanishing gradient are viewed as milestones and key-moments of the history of both machine learning and Deep Learning. Sepp Hochreiter laid the foundations for Deep Learning in two ways. Dr. Hochreiter’s seminal works on the vanishing gradient and the Long Short-Term Memory (LSTM) were the starting points for what became later known as Deep Learning. LSTM has been overwhelmingly successful in handwriting recognition, generation of writings, language modeling and identification, automatic language translation, speech recognition, analysis of audio data, as well as analysis, annotation, and description of video data. Sepp Hochreiter is full professor at the Johannes Kepler University, Linz, Austria and head of the Institute for Machine Learning. He is a German citizen, married and has three children.
Title: Memory Concepts for Large Language Models
Memory Concepts for Large Language Models
Currently, the most successful Deep Learning architecture for large language models is the transformer. The attention mechanism of the transformer is equivalent to modern Hopfield networks, therefore is an associative memory. However, this associative memory has disadvantages like its quadratic complexity with the sequence length when mutually associating sequences elements, its restriction to pairwise associations, its limitations in modifying the memory, its insufficient abstraction capabilities. The memory grows with growing context. In contrast, recurrent neural networks (RNNs) like LSTMs have linear complexity, associate sequence elements with a representation of all previous elements, can directly modify memory content, and have high abstraction capabilities. The memory is fixed independent of the context. However, RNNs cannot store sequence elements that were rare in the training data, since RNNs have to learn to store. Transformer can store rare or even new sequence elements, which is one of the main reasons besides their high parallelization why they outperformed RNNs in language modelling. I think that future successful Deep Learning architectures should comprise both of these memories: attention for implementing episodic memories and RNNs for implementing short-term memories and abstraction.
Date: February 7, 2024
Time: 11:30
Place: Universitätstraße 32.101, Campus Vaihingen of the University of Stuttgart.
Looking forward to seeing you all there! No registration necessary.